Dr. Kate Bentley discusses studies in suicide prevention using new technologies
March 31, 2023
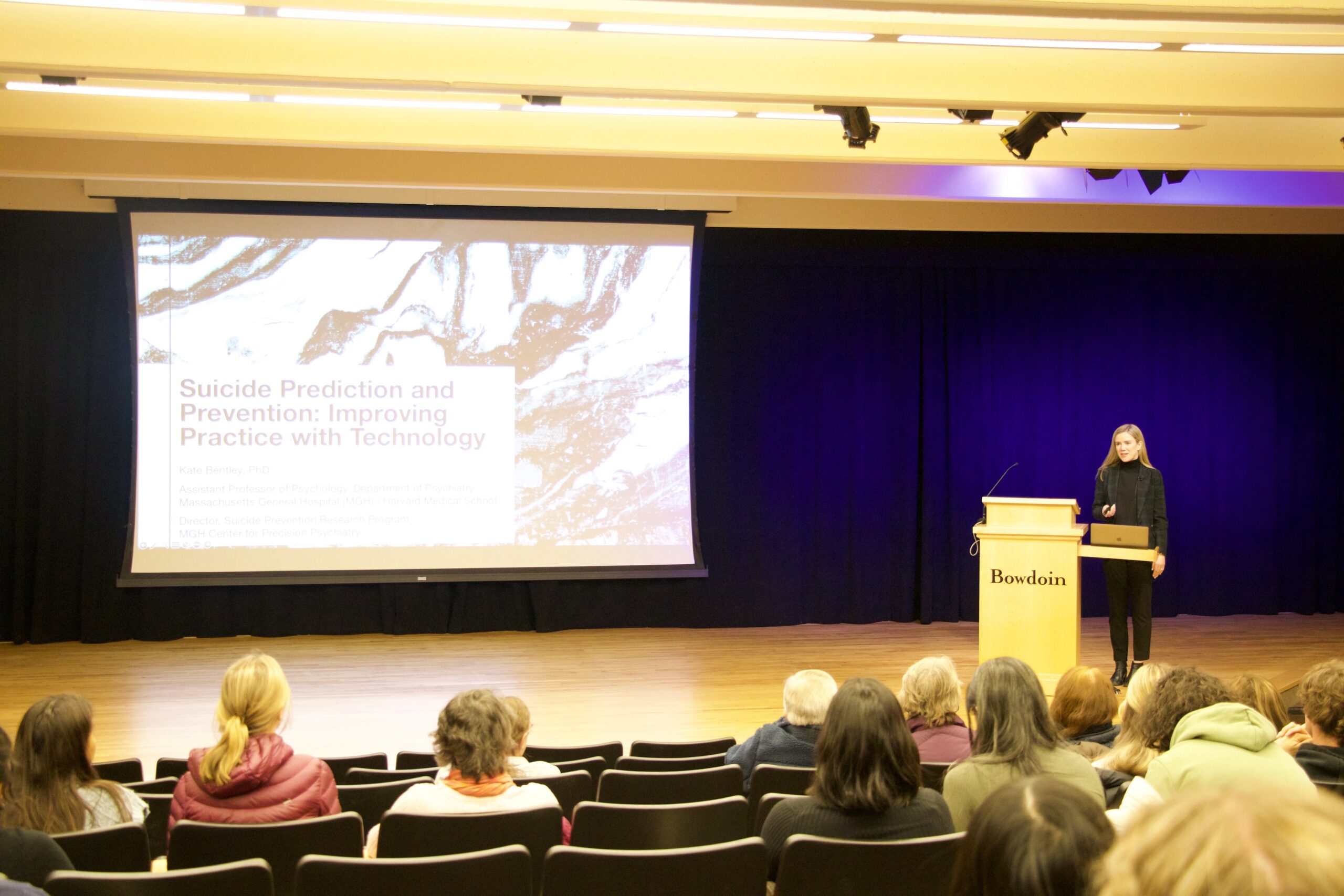
On Tuesday evening, the Department of Psychology invited Harvard Medical School assistant professor and Massachusetts General Hospital clinical psychologist Dr. Kate Bentley to campus. In her lecture, Bentley presented her ongoing research on identifying risk factors for suicide and using technology to predict and prevent suicide attempts.
Bentley acknowledged that elevated stress levels and anxiety in college students have likely contributed to recent increases in suicide rates among young adults. Yet, despite rising demand for mental health care on campuses nationally, rates of treatment seeking remain low. Accordingly, a key focus of Bentley’s lab is to develop accessible and destigmatized interventions for suicide prevention.
“My team and many others are doing work to try to improve access to [suicide] interventions and also improve how effective these existing interventions are, especially during really critical, high-risk moments,” Bentley said.
Bentley explained that a major step in enhancing suicide prevention measures is identifying risk factors. However, even with the vast research conducted, little progress has been made toward using these factors to accurately predict and prevent suicide.
To address this discrepancy, Bentley’s lab used machine learning to build statistical models that use a patient’s electronic health records such as diagnoses, treatment history, demographic characteristics, age, sex and ethnicity to predict an individual’s risk for suicide.
“The advantages of these types of machine learning models over much of the existing work that I’ve described earlier is that they can incorporate vast amounts of healthcare data,” Bentley said. “Another really important thing about these machine learning models is that they don’t rely on patients [to] self-report, meaning a person does not need to disclose that they’re having thoughts of suicide.”
In addition to health record assessments, Bentley believes that real-time monitoring and assessments could provide the urgent support needed to aid individuals showing acute and imminent signs of suicide. These response-based techniques are known as “Just In Time Adaptive Interventions” (JITAIs).
“JITAIs involve delivering messages or prompts, perhaps through smartphone[s] or another mobile device, that are timely and that are triggered by real-time mobile data collected over time from a person,” Bentley said.
Physiological conditions, movement, surveys, communication patterns and location are all examples of real-time information that researchers observe over a short period of time.
“For example, smartphone surveys could show a certain pattern of responses that are concerning, or certain physiological signals that might be picked up from a wearable device to show that someone’s in distress and triggers an intervention.”
Other studies conducted by Bentley’s lab utilized quick self-report surveys sent out multiple times a day through an app to gauge a person’s emotions and provide specific skills to reduce a patient’s suicidal urges. Common indicative risk factors like anxiety, agitation, shame and self-hatred were significantly reduced over time after patients implemented the suggested skills in this study.
“It’s one thing to learn a coping skill in a session with a therapist. It’s quite another thing to remember to try that coping skill when you’re in distress and remember how to use it effectively,” Bentley said. “Smartphone apps and other sorts of digital interventions may provide a real opportunity to help people during these high-risk moments when they most need skills.”
However, this research poses several ethical questions about the use of machine learning and the collection of real-time patient data to assist suicidal patients. Furthermore, Bentley recognized the potential bias of statistical models to predict behavior and recommend interventions in high-risk situations. Bentley hopes to address some of these ethical concerns through future studies.
Jasmine Jia ’25 also expressed some of her concerns with Bentley’s app-based approach to treatment, especially among college students who may be directed to this method as a substitution for in-person care.
“Many mental health crises or emotional emergencies happen at a more acute rate. A threshold to seek professional health at suicidal levels isn’t very helpful to a lot of the population, who genuinely need to see a professional therapist and may find this method impersonal or ineffective,” Jia said.
Associate Professor of Psychology Hanna Reese organized the lecture and commented on the significance of bringing Bentley to campus, along with her takeaways from the event.
“I was so encouraged by the number of students in attendance [of the lecture]. As Dr. Bentley showed in her talk, suicidal thoughts and behaviors are more common than many people realize, especially among college students,” Reese said. “A critical component of suicide prevention is being willing to have a conversation about it.”
Comments
Before submitting a comment, please review our comment policy. Some key points from the policy: